The Dangers Of Dirty Data
Is your organisation working with ‘dirty data’? How would you know? And, what impact is it having? This article has everything you need to know about doing a quick spot check, spotting procurement problems, identifying savings, and more importantly, making sure your data has its COAT on.
We all think we know what dirty data is, but it can mean very different things depending on who you speak to. At its most basic level, dirty data is anything incorrect. In detail within procurement, it could be misspelled vendors, incorrect Invoice descriptions, missing product codes, lack of standard units of measure (e.g. ltr, L, litres), currency issues, duplicate invoices or incorrect/partially classified data.
Dirty data can affect the whole organisation, and we all have an impact on, and responsibility for the data we work with. Accurate data should be everyone’s responsibility, but currently across many organisations data is the sole responsibility of a person or department, and everyone trusts them to make sure the data is accurate.
But, they tend to be specialists in data, analytics and coding, not procurement. They don’t have the experience to know when a hotel should be classified as accommodation or as venue hire, or what direct, indirect or tail spend is and its importance or priority.
How many times have you been working with a data set and noticed a small error but not said anything, or just manually corrected something from an automated report, just get it out the door on time? It feels like too much of an inconvenience to find the right person to notify, so you just correct the error each time yourself, or you raise a ticket for the issue but never get round to checking if it’s resolved.
These small errors that you think aren’t that important can filter all the way up to the top of an organisation through reports and dashboards where critical decisions are being made. It happens almost every day.
How does this affect my organisation?
There are many ways, but one of the most widespread and noticeable impacts is around reporting and analytics. If you’re in senior management, you will most likely receive a dashboard from your team that you could be using to review cost savings, supplier negotiations, rationalisation, forecasting or budgets.
What if within that dashboard was £25k of cleaning spend under IBM? I can already hear you saying “that’s ridiculous” – well, it is obvious when pointed out, but I have seen with my own eyes IBM classified as cleaning. It can happen easily and occurs more frequently than you might think.
Back to that dashboard that you are using to make decisions, you’ll see increased spend in your cleaning category, and a decrease in your IT spend, which could affect discounts with your supplier, your forecast for the year, monitoring of contract compliance etc… It could even affect reporting of your inventory, it appears you need more laptops, and unnecessary purchases are made.
When there are tens or hundreds of thousands of rows of data, errors will occur multiple times across many suppliers. And for the wider organisation, this could affect demand planning, sales, marketing and financial decisions.
And then there are technology implementations. Rarely is data preparation considered before the implementation of any new software or systems, and there can even be the assumption that the software supplier will do this, which may not be the case, and if they do provide that service it might not be good enough.
It can be very far into the process of implementation before this is uncovered, by which time staff have lost faith in using the software, are disengaged, claim it doesn’t work, or they don’t trust it because “it’s wrong”.
At this point, it either costs a lot of money to fix and you have to hope staff will engage again, or the project is abandoned. In either case, this can take months and cost thousands, not millions of pounds/euros/dollars in abandoned software or reparation work.
You might also be considering using, or engaging with a 3rd party supplier that uses AI, machine learning or some form of automation. I can’t emphasise enough the importance of cleansing and preparing your data before using any of these tools.
Think back to the IBM example, each quarter the data is refreshed automatically with the cleaning classification, that £25k becomes £50k, then £75k the following quarter, it’s only when the value becomes significant that someone notices the issue. By this stage, how many decisions have been based on this incorrect information?
How can this be resolved?
Truthfully, it’s with a lot of hard work. There’s no magic bullet or miracle solution out there to improve the accuracy of your data: you have to use your team or an experienced professional to get the job done. Get your team to familiarise themselves with the data. If they are reviewing and maintaining it regularly they will soon be able to spot errors in the data quickly and efficiently.
If you think about data accuracy in terms of COAT, this will help to manage your data.
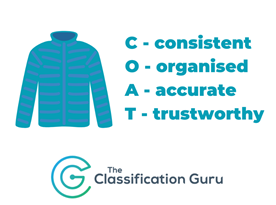
It should always be Consistent – everyone working to the same standards; Organised – categorised properly; and Accurate – correct. And only when you have these things will it also be Trustworthy – you wouldn’t drive around in a car without a regular inspection would you?
How to spot procurement problems and identify savings
Accurate data is important, but in its raw state, it’s not the whole story. As a procurement professional you’re tasked with ensuring the best prices for products or services, as well as ensuring contract compliance on those prices, along with cost reductions and monitoring any maverick spend … to name but a few!
Accurate data alone will not help achieve this, I strongly recommend supplier normalisation and spend data classification to help quickly and efficiently manage spend and suppliers, monitor pricing and spot any potential misuse of budgets.
How do I get started?
With a spreadsheet of spend transactions over a period of time such as 12 to 24 months, the first step should be Supplier Normalisation, where a new column is added to consolidate several versions of the same company to get a true picture of spend with that one supplier. For example, I.B.M, IBM Ltd, I.B.M. would all be normalised to IBM.
Data can be classified using minimum information, such as Supplier Name, Invoice/PO line description and value. To get more from the data, other factors can then be added in, such as unit price. Where unit price information is not available, the quantity can be divided by the overall value.
A suitable taxonomy will then need to be found to classify the data. It can be an off the shelf product such as ProClass, UNSPSC, PROC-HE, or a taxonomy can be customised so it’s specific to your organisation or industry.
This initial stage may take months if you are working with large volumes of data. It might be worth considering outsourcing this initial task to professionals experienced in this area, who will be able to complete the project in a shorter time, with greater accuracy.
Avoiding common pitfalls
There are a number of ways to classify the data> However, to get started, look for keywords in the Supplier Name and then the Description column. The description of services could include ‘hotel, taxi, cleaning services, cleaning products, etc., however, it’s important to carefully check the descriptions before classifying, or errors could be introduced. A classic example is “taxi from hotel to restaurant”, depending on which keyword you search for first, it could end up being misclassified as transport, or venue costs.
I wouldn’t advise classifying row by row, as it could take more than twice as long to complete the file using this method. Start with keywords, followed by the highest value suppliers which you can get from a pivot table of the data if you’re working in Excel.
Identifying opportunities
Once classified, charts can be built to analyse the data. The analysis could include, ‘top 80% of suppliers by spend’; ‘number of suppliers by category’; ‘unit price by product by month’; ‘spend by category’; or ‘spend by month.’
Patterns should start to emerge which could reveal unusually high or low spend in a category, irregular pricing, higher than expected use of services, or a higher than expected number of suppliers within a category.
Why you should strive for data accuracy and classification?
Data accuracy is an investment, not a cost. Address the issues at the beginning: while it might seem like a costly exercise, you will undoubtedly spend less than if you have a to resolve an issue further down the line with a time-consuming and costly data clean-up operation. And by involving the whole team or organisation, it will be much easier to manage and maintain the most accurate data possible.
Spend data classification shows you the whole picture, as long as it’s accurate. You can get a true view of your spend, allowing improved cost savings, better contract compliance and possibly the most important – preventing costly mistakes before they happen.
So, does your data have its COAT on? What does ‘dirty data’ mean to you? Let me know below!
Susan Walsh is the founder of The Classification Guru, a specialist in spend data classification, supplier normalisation and taxonomies. You can contact her at [email protected] https://www.procurious.com/professionals/susan-walsh